Quant²AI
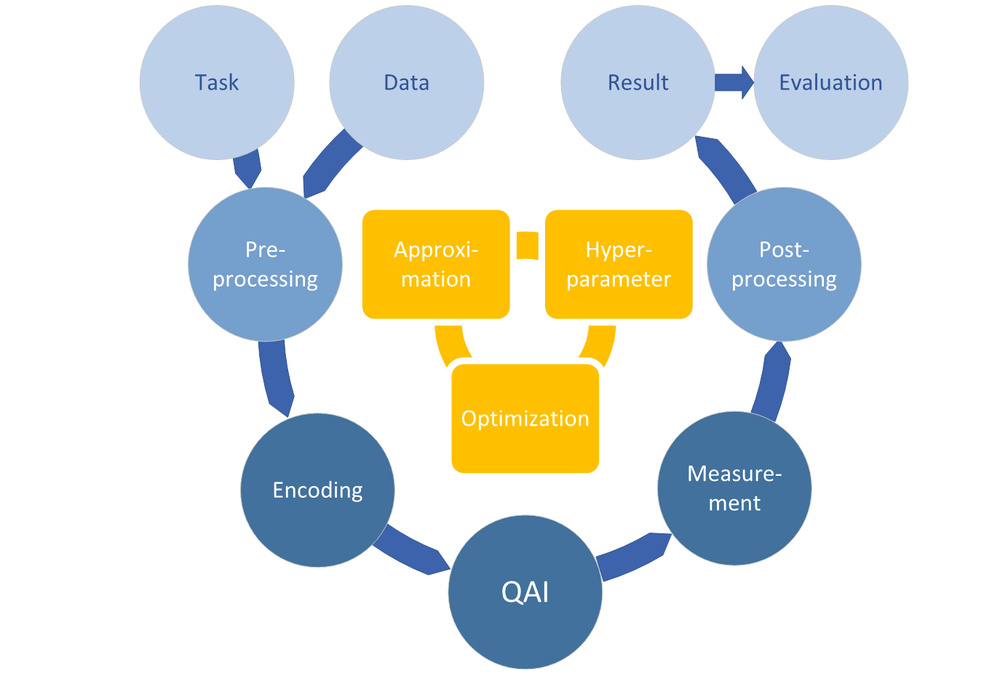
Assessing Quantum AI
Quantum computers work according to computational principles from quantum physics that are very different from classical computers. This gives rise to the hope of achieving immense advantages over current computing approaches - especially for resource-intensive applications such as artificial intelligence. The Institute for AI Safety is investigating whether these hopes can be realised from a heuristic point of view in the project Quantification of Quantum Advantages for Artificial Intelligence. In this project, which is funded by the DLR Quantum Computing Initiative, we will work on the application-oriented evaluation of quantum AI pipelines until the end of 2025. This will make it easier for users to make a qualified decision in favour of a method and to assess the expected benefits. In addition to the Institute for AI Security, Fraunhofer ITWM, Conet, Data Cybernetics and Jos Quantum are involved in the project as contractors.
Contribution of the Institute for AI Safety and Security
The applications for artificial intelligence are constantly expanding, whether it is controlling autonomous systems or recognising patterns in images. This increases the demands on AI. However, optimising an AI model is a complex process that requires large amounts of data to be analysed using large amounts of computing power. Many stakeholders expect the transition to quantum AI to improve the learning process, among other things. These expectations are often not clearly articulated and not supported by much evidence: For current quantum AIs, there are mostly only feasibility studies, which look at individual sub-steps and are difficult to compare.
Quant²AI adds quantitative insights to the discourse on the potential benefits of different quantum AI approaches. In a first step, we systematise possible benefits and map the performance of an AI system into a suitable metric. Together with a set of application-oriented example problems, this will lead to a benchmark, a prototype of which will be available by the end of the project. This will cover the entire process, from preparing and coding the data sets to interpreting the results. This is necessary because gains from the use of quantum computers in individual sub-steps will only bring relevant advantages if they can also be incorporated into the AI pipeline without losing performance gains elsewhere, for example due to the need for complex data coding.
The data sets cover DLR's core areas such as aerospace, transport and energy. In addition, the industrial companies contribute further topics from the fields of materials science and finance. We use this data to evaluate the trained AI models in terms of metrics for expected quantum advantages.
Quant²AI gives users the ability to choose between different quantum AI methods for their use case and to realistically assess possible advantages over classical methods. Developers get a tool to evaluate their algorithms and identify potential bottlenecks. Quant²AI thus creates an overview and comparability for newly developed quantum AI methods.