Image Mining
In DLR’s Earth Observation Center (EOC), petabytes of satellite image data are processed, stored and administered, presenting new opportunities and challenges, such as how to exploit this big data.
This prompted us to look for image information mining (IIM) systems capable of extracting information and presenting it in a way understandable to human users. An IIM system is composed of machines (performing based on a machine learning algorithm), users, and all the interactions occurring between the users and machines, the different machines, and the different users. In spite of great advances, the results of most existing IIM systems are not satisfactory to users. For example, when users search for a semantic label (e.g., “house”), they might retrieve very diverse results which don’t always match their mental representation of the term. This can be due to other users’ mental representations which were transferred to the machine as they trained it on their own understanding of the term.
This dissatisfaction is caused by the sensory and semantic gaps. The sensory gap refers to the difference between object perception with the naked eye, and the perception of the object based on the images created from sensor recorded signals. The semantic gap is defined as the difference in the understanding of objects in an image between users and machines, as well as the differences in image understanding between various users.

Most past literature has taken a computational approach to deal with the semantic gap, addressing different aspects of the machine, such as the learning algorithms being used. In this approach, the users and their interactions within the system are not considered.
We address this problem of user dissatisfaction with an interdisciplinary approach, combining findings and methods from the fields of Computer Vision and Cognitive Psychology. In our research, we conduct user experiments to quantify the sensory and semantic gaps, as well as finding ways to reduce them.
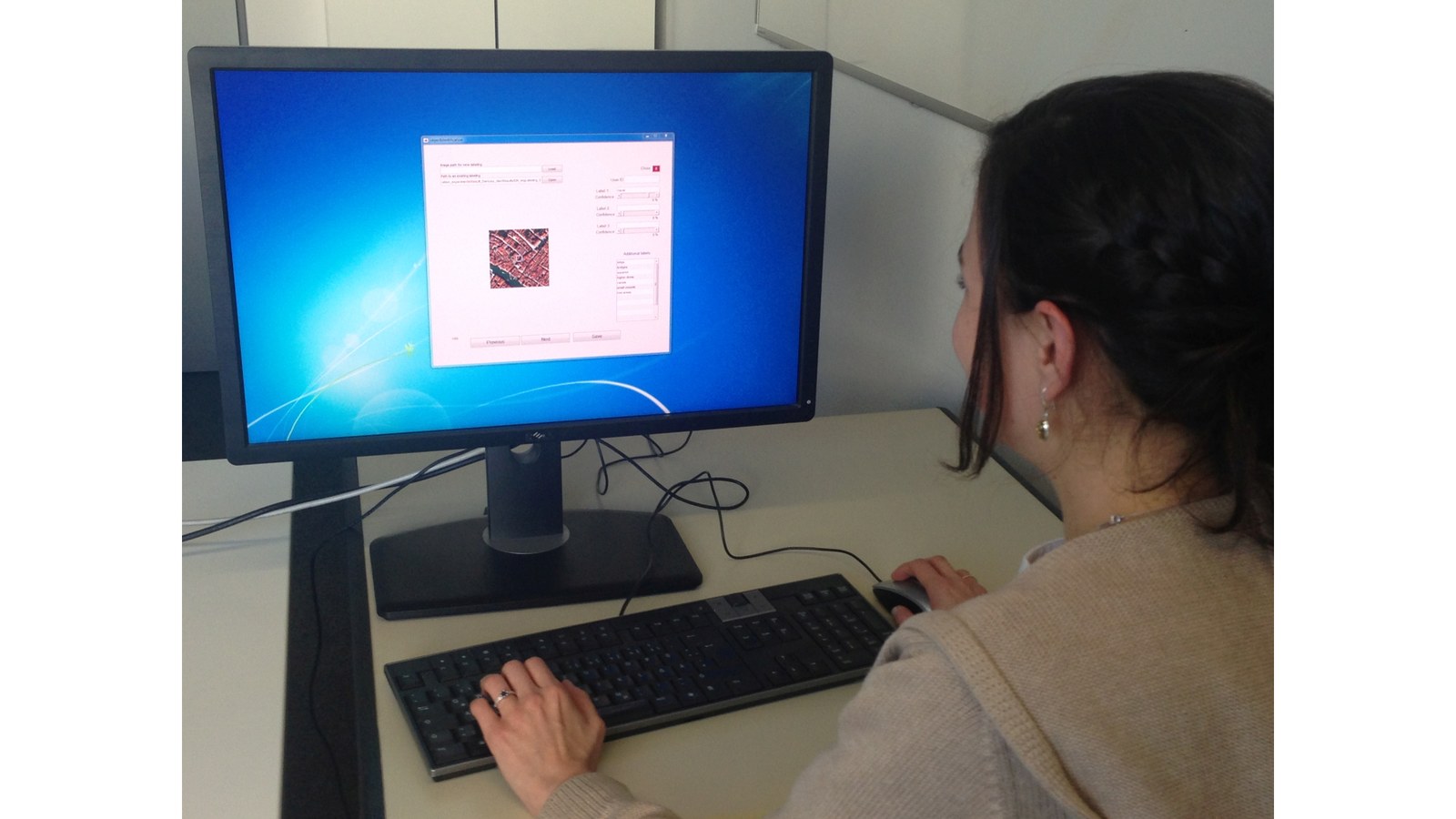