Embed2Scale
Technically, Embed2Scale is going to advance federated remote sensing analytics with the aid of self-supervised learning for data-driven compression and representation across data centers. Multi-modal deep learning foundation models are at the heart of our data-driven approach. Downstream tasks range from weather modeling to maritime awareness.
The past decade witnessed a remarkable success of data science to master human tasks as recently demonstrated by generative models such as ChatGPT. While remote sensing streams petabytes of optical, radar, and LiDAR data for various Earth observation applications, human labeling and efficient multi-modal data fusion to train deep neural networks poses a major challenge.
The MF-DAS team “Large-Scale Data Mining in Earth Observation” (DM4EO) will advance the boundaries of scalable, weakly supervised learning with the aid of geospatial foundation models. We aim to research federated deep learning across high-performance compute infrastructure in collaboration with academic, government, and corporate partners - among others: Oxford University, Juelich Supercomputing Center, IBM Research, the European Union Satellite Center, and Sinergise/Planet. Embed2Scale further naturally integrates with DLR projects such as terrabyte.
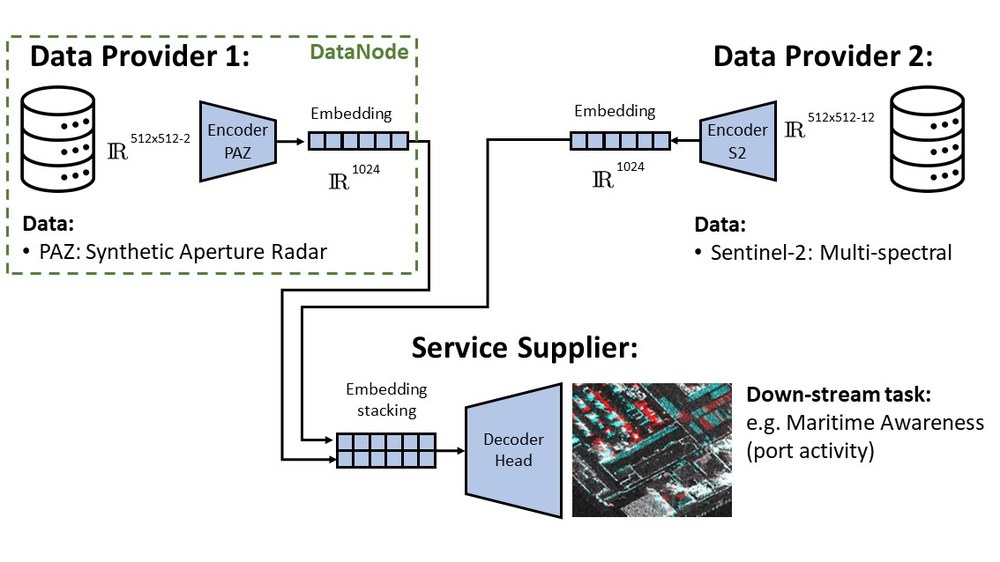