Quantum reinforcement learning
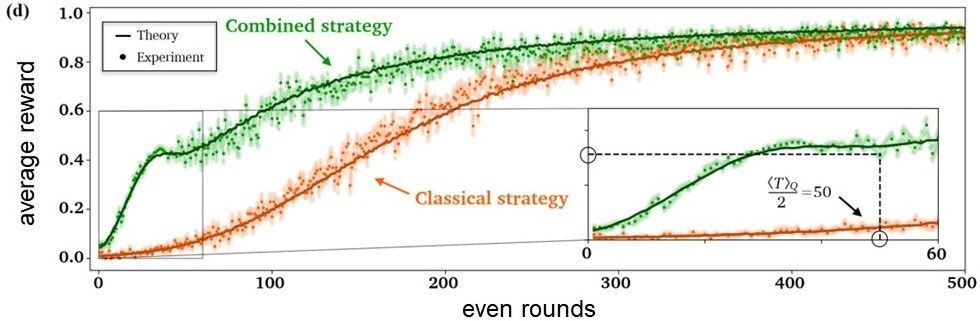
Copyright: Springer Nature, Quelle: Nature 591, 229 (2021)
Humans learn permanently new stuff by exploring things and exploiting the obtained experience. Machines, so-called learning agents, can also learn by reinforcing the execution of actions which have led to rewards in the past. However, it usually takes time to find rewarded actions and thus to learn. Quantum algorithms can speed up this search for rewarded actions.
We demonstrated in a proof-of-principle experiment such quantum-enhanced learning together with the Universities of Vienna, Innsbruck, Leiden and the MIT. In the performed experiment, a quantum-enhanced agent learned to solve an abstract problem within 100 attempts on average. In contrast, a corresponding classical agent needed 266 attempts on average.
However, there still exist many challenges which need to be solved in order to apply quantum reinforcement learning to real problems. On the one hand, only simple quantum algorithms can be implemented so far with existing quantum computers. On the other hand, we have to consider the principles of quantum mechanics, such as the wave-particle dualism, when developing learning strategies for different problems. A quantum agent can explore different actions at the same time similar to water waves which spread towards all directions. This phenomenon is called superposition. However, superposition collapses when we measure which action the agent has performed and whether it obtained some reward. Therefore, it is important to find optimal strategies to combine quantum exploration and classical exploitation in order to solve real problems.