What? How? Why? Explainable artificial intelligence in robotics
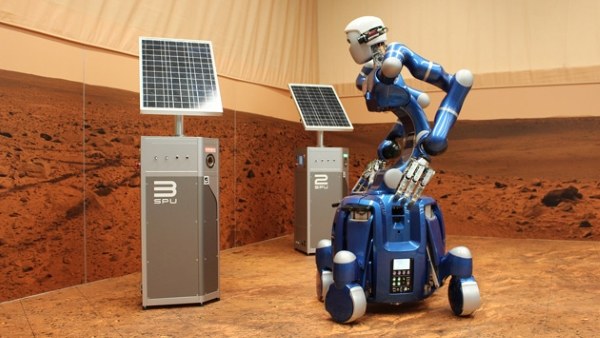
How much do the robots at the DLR Institute of Robotics and Mechatronics know about what they do and why? To find out, Freek Stulp - Head of the Department of Cognitive Robotics - has launched the "Green Button Challenge". He reports on his experiences on the DLR blog:
Robotics is closely linked to the topic of artificial intelligence (AI). After all, robots are built to do something - and to know what to do and how, they need a certain amount of intelligence. The great interest in AI in recent years has mainly been generated by data-driven methods, especially by so-called "deep learning". Breakthroughs in this area - for example, their successful application in speech or image recognition - have been made possible because large amounts of data and more computing capacity are now available.
Such deep learning methods recognize for example a solar panel or a spoon in an image because they extract patterns from the image that are typical for solar panels or spoons. However, recognition in itself does not mean that a robot knows that spoons are used for eating - nor how best to grip spoons to use them. For a robot on the Moon or Mars, it is not only useful to recognize solar panels, but also to know that it can charge its battery there. A robot that does not know the function of objects cannot know what it is doing.
The "Green Button Challenge"
To find out which robots at the Institute
for robotics and mechatronics know what they do and why, I have set my department for cognitive robotics a challenge: Within a week, a green button should be available next to selected robots. When I press this button, the robot should explain to me what it is doing. And when I press it again, I want to hear it explain why. Five teams have accepted this "Green Button Challenge". For some robots, knowledge of what they are doing was already relatively well represented in the computer program. It just needed to be "spoken". With other robots the knowledge was rather implicit and inconsistent. In these cases, the programmers had told the robot how to do something (for example, exact movements needed to grip a bottle), but had not explicitly described in the program what to do or why (for example, that the bottle is gripped and then water is poured into a cup). So programming the "what" and "why" of these robots was more work. After a week of intensive thinking and programming, the individual teams presented their Green Button solution.


To ensure that all robots were able to pronounce the generated sentences, one of the teams implemented a deep learning approach for this and made it available to all other teams. Deep learning is unbeatable for this type of task.
The robots begin to explain
At first, it was unusual and impressive for us researchers, too, that our robots literally started to explain what they were doing at the push of a button. For example, one of the robots hardly stopped chattering about one of its joints! In the end, the team around our Rollin' Justin won the competition because Justin was able to report in detail and in many different ways on what he was doing and what his goal was.
For example, Justin was busy rotating a solar panel when someone pressed the green button. "I want to execute action rotate solar panel 1," explained Justin. Next button press: "Because I want to have solar panel 1 in a different orientation" - button press - "Because I want to execute action clean solar panel 1" - button press - "Because I want to have a clean solar panel". Freely translated: "I want to turn the solar panel because it should have a different orientation; because I want to make it clean, because my goal is to have a clean solar panel". That sounds very reasonable: Justin can explain what he's doing. By the way, this is exactly the task Justin performed for Alexander Gerst when the astronaut remote-controlled the robot in 2018 from the International Space Station (ISS) - as part of the METERON project.

Your consent to the storage of data ('cookies') is required for the playback of this video on Youtube.com. You can view and change your current data storage settings at any time under privacy.
The last time Justin pressed the button, he said, "I don't know. Nobody told me". Now Justin can no longer explain his actions. He was charged with achieving the goal of "clean solar panel". He knew what to do and how to do it. But he doesn't know the background to the assignment.
This last example shows where the limits lie. Our robots do not set their own goals. And when the lights go out in the lab at night, they don't ask themselves questions like "Why do I exist?" This is not technically feasible and we do not want to achieve it. Robots should not have existential crises, but should support us in tasks in space and on earth. However, if a robot can explain to us in a simple and plausible way what it is doing at the moment, then we can understand if and how it achieves the given goals.
In addition, my goal in the Green Button Challenge was to create awareness and understanding among the employees in my department that robots often do not even know what they are doing - let alone are capable of communicating it. In AI research, this is called the "explainability" of a system, also called "Explainable Artificial Intelligence" or "XAI". But this explainability is essential if we are to interact with robots and trust them: this is the so-called "Trustworthy Artificial Intelligence". Do you want to work with someone who has no idea what he is doing - or who can't explain it to you?
An unresolved question with a long history
In the early days of AI in the 1950s, one of the first big questions was how to represent knowledge in intelligent systems. Although the question has by no means been conclusively answered, this discussion has unfortunately fallen somewhat into oblivion today - partly because of the fuss about deep learning. But I am convinced that data-driven methods can do little to answer this question. For me, robotics is so exciting because it requires a new edition and revival of the question of knowledge representation.
On the one hand, it is highly philosophical: If you can explain and pronounce your actions - like Justin is now - does it mean that you know what you are doing? Or does one need consciousness - which Justin certainly does not have - to really know something? Apart from these philosophical questions, I also find robotics fascinating from a pragmatic point of view, because our AI research is directly relevant to real fields of application: from space assistance (as in the METERON project), to robots in care, to the factories of the future.
Of course, we don't claim to have answered an almost 70-year-old question in seven days. The topics of knowledge representation and explainability are still prominent on our research agenda, even beyond the green button. Now that the competition is over, we are working together and with the other departments of the institute to look at, understand, and integrate the various solutions.
Already today, I cordially invite you to our public events so that our robots can explain what they are doing - at the push of a button!