News
Loading
At DLR
Loading
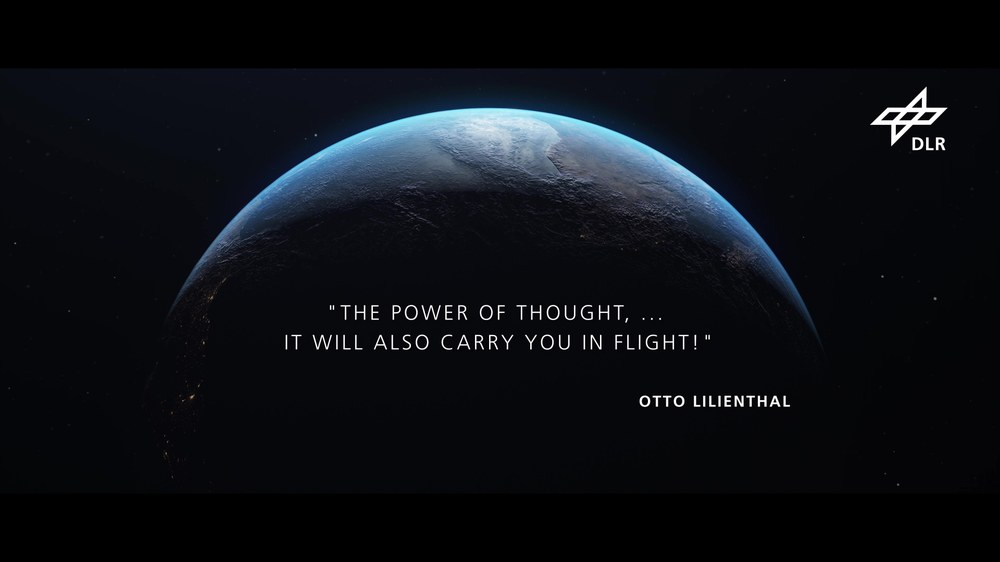
Video:
DLR at a glance
Your consent to the storage of data ('cookies') is required for the playback of this video on Youtube.com. You can view and change your current data storage settings at any time under privacy.
DLR at a glance
Your consent to the storage of data ('cookies') is required for the playback of this video on Youtube.com. You can view and change your current data storage settings at any time under privacy.